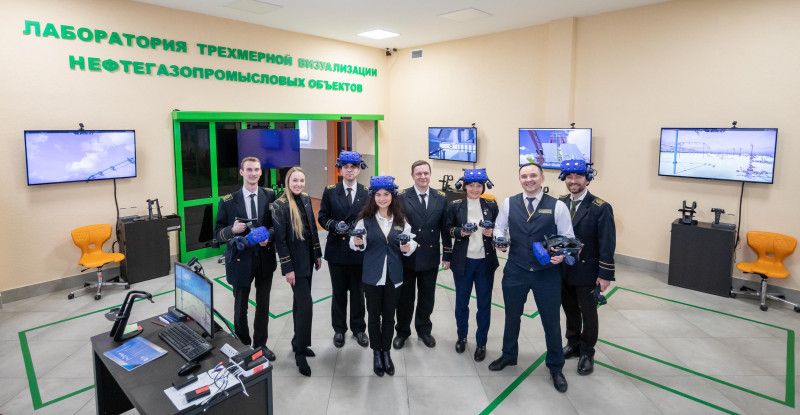
Cкорость научно-технологического прогресса и исчезновение определенных видов деятельности, связанное с проникновением автоматизации во все сферы производственных и управленческих процессов, являются факторами возможного роста для предприятий будущего. Цифровая интеграция, объединяющая научные направления, кадры, процессы, пользователей и данные, будет создавать условия для научно-технических достижений и прорывов, обеспечивая научно-экономические сдвиги в смежных отраслях и, прежде всего, на глобальном минерально-сырьевом рынке. В этой связи в 2018 году с целью обучения, исследований и разработок в области цифровых технологий для предприятий минерально-сырьевого и топливно-энергетического комплексов в Горном университете создан «Образовательный центр цифровых технологий».
Подробнее о задачах
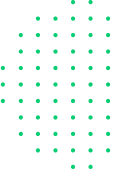
Направления научных исследований
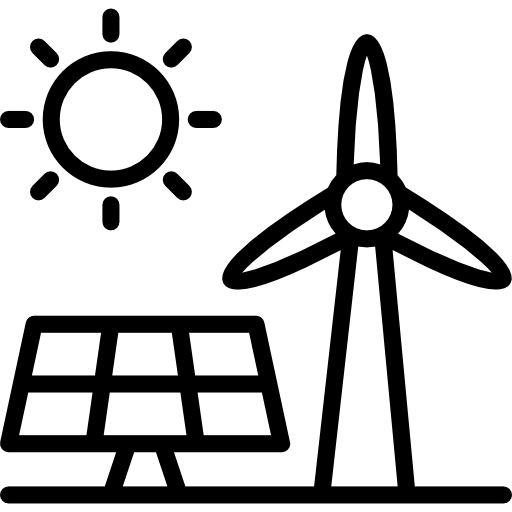
Исследование эффективного развития и функционирования энергетических систем на новой технологической основе, принципах энергосбережения, современной электротехнике, ВИЭ
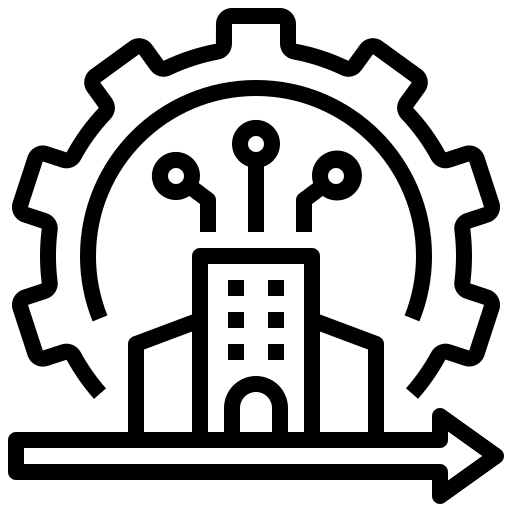
Теория и методология информационного обеспечения объектов недропользования
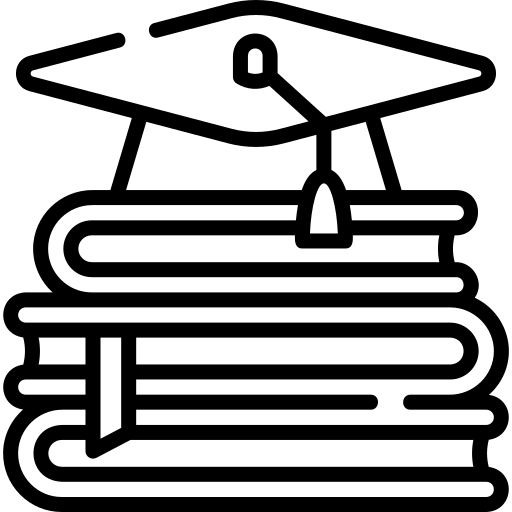
Создание системы непрерывного обучения и повышения квалификации, направленной на формирование профессиональных цифровых компетенций специалистов, необходимых для обеспечения инновационного развития ТЭК и МСК
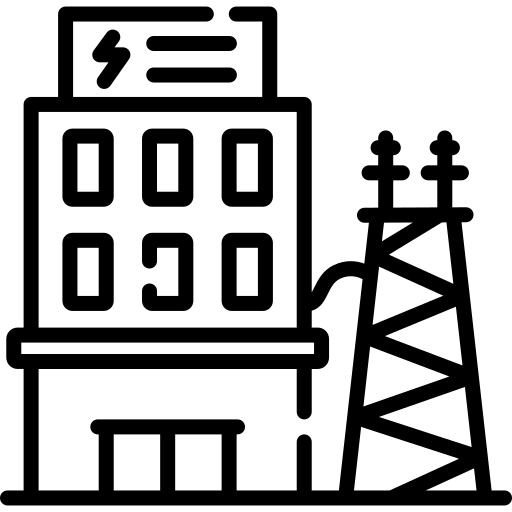
Энергосбережение и повышение энергетической эффективности
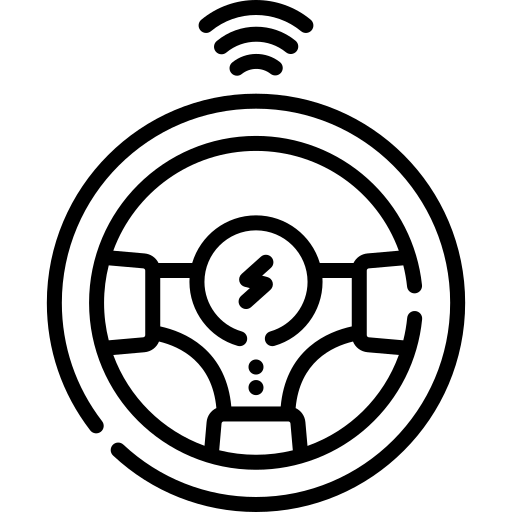
Переход к передовым цифровым, интеллектуальным производственным технологиям, роботизированным системам на предприятиях МСК и ТЭК
Данное направление предполагает рассмотрение интеллектуальных технологий управления электроэнергетическими системами, включая передачу электрической энергии, управление спросом на электрическую энергию, цифровые двойники объектов электроэнергетики, цифровые информационные модели электротехнических систем.
Читать далее
Лаборатория
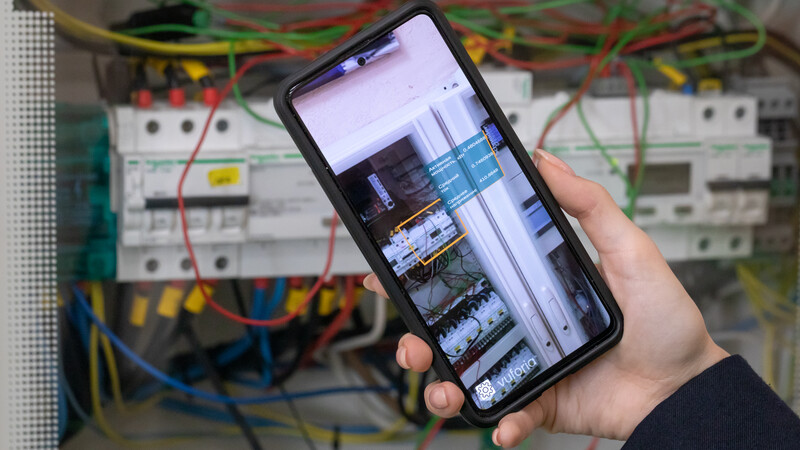
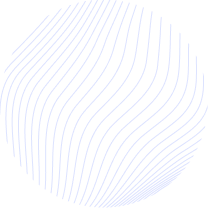
В рамках данного направления ведется разработка новых методов мониторинга и управления на основе цифровых и информационных технологий, создание информационных систем для решения задач горной отрасли.
Читать далее
Лаборатория
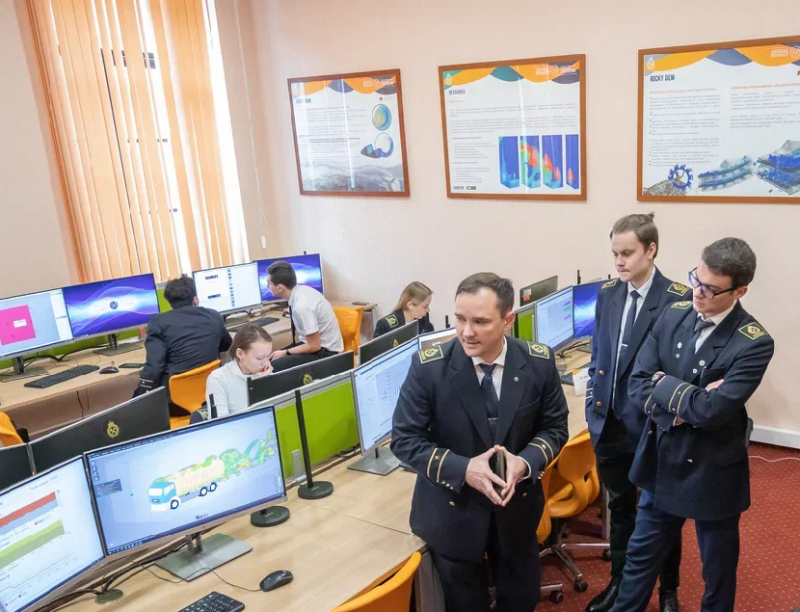
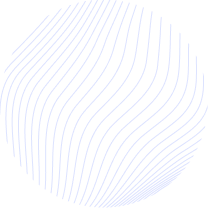
Данное направление нацелено на развитие и популяризацию инженерного образования, повышение цифровых компетенций сотрудников и обучающихся, а также реализацию программ дополнительного профессионального образования для представителей компаний ТЭК и МСК.
Читать далее
Лаборатория
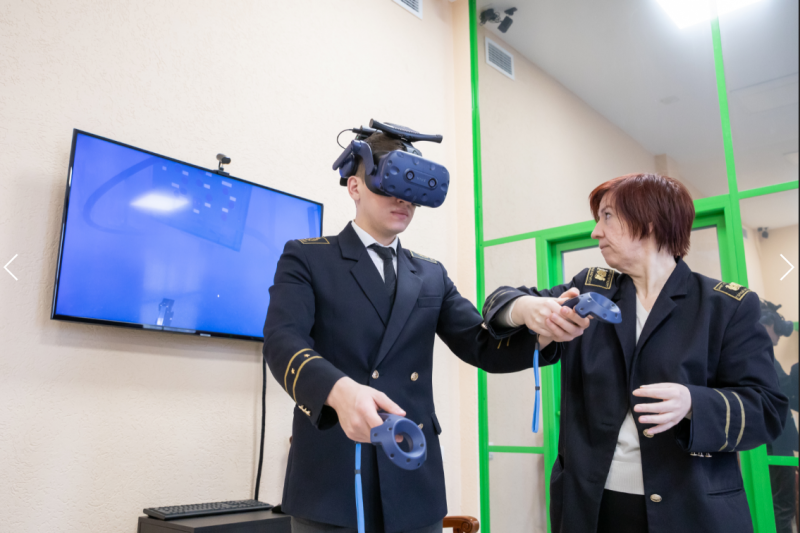
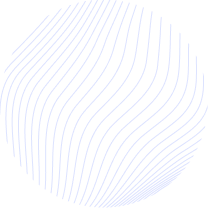
Данное направление предполагает исследование и обоснование комплексных показателей эффективности генерации, транспорта и потребления энергии при снабжении от традиционных и возобновляемых источников энергии с учетом влияния глобальных вызовов и вариации внешних факторов.
Читать далее
Лаборатория
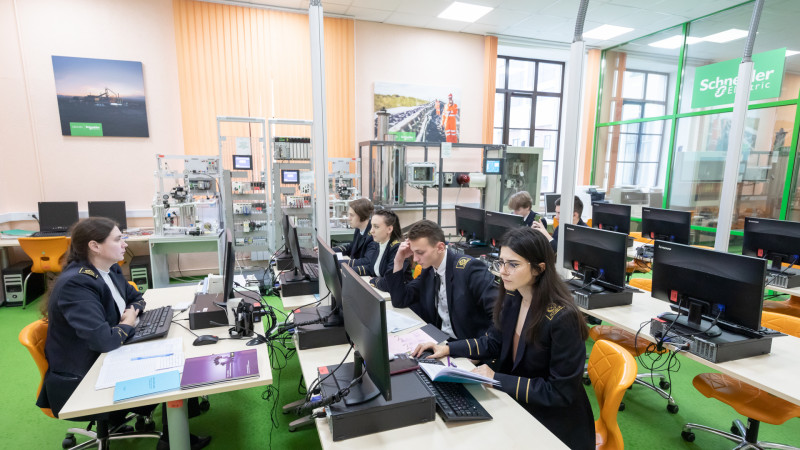
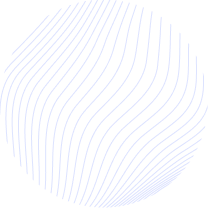
В рамках данного направления проводятся исследования, направленные на повышение эффективности оборудования и технологических процессов добычи, переработки и транспортировки полезных ископаемых.
Читать далее
Лаборатория
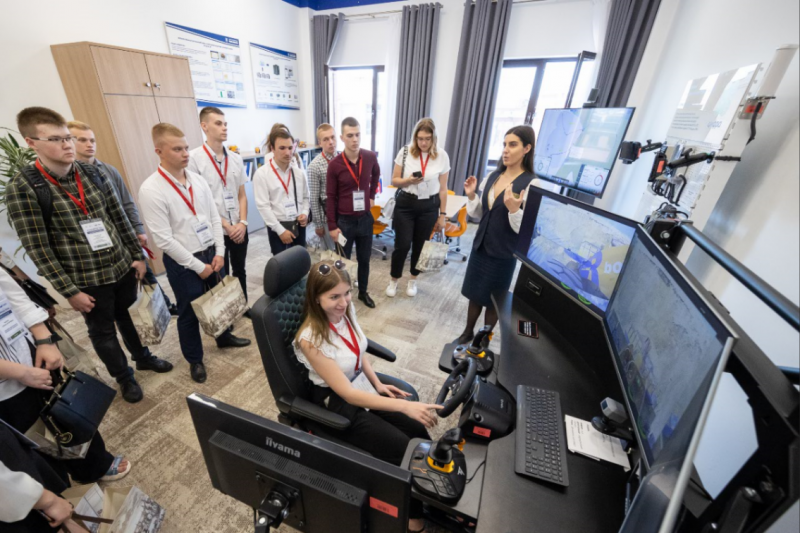
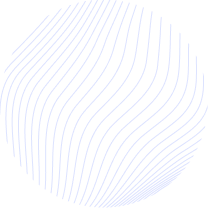
Проекты
Научные публикации

Method of Data storing, collection and aggregation for definition of life-cycle resources of electromechanical equipment
Дата публикации: 2017-10-20
Журнал: IOP Conference Series: Earth and Environmental Science
Авторы: Zhukovskiy, Y, Koteleva, N.
ISSN:17551315
Analysis of technical and technological conditions for the emergence of emergency situations during the operation of electromechanical equipment of enterprises of the mineral and raw materials complex shows that when developing the basis for ensuring safe operation, it is necessary to take into account not only the technical condition, but also the non-stationary operation of the operating conditions of equipment, and the nonstationarity of operational operating parameters of technological processes.
Violations of the operation of individual parts of the machine, not detected in time, can lead to severe accidents at work, as well as to unplanned downtime and loss of profits. That is why, the issues of obtaining and processing Big data obtained during the life cycle of electromechanical equipment, for assessing the current state of the electromechanical equipment used, timely diagnostics of emergency and pre-emergency modes of its operation, estimating the residual resource, as well as prediction the technical state on the basis of machine learning are very important.
This article is dedicated to developing the special method of data storing, collection and aggregation for definition of life-cycle resources of electromechanical equipment. This method can be used in working with big data and can allow extracting the knowledge from different data types: the plants' historical data and the factory historical data. The data of the plants contains the information about electromechanical equipment operation and the data of the factory contains the information about a production of electromechanical equipment.
Violations of the operation of individual parts of the machine, not detected in time, can lead to severe accidents at work, as well as to unplanned downtime and loss of profits. That is why, the issues of obtaining and processing Big data obtained during the life cycle of electromechanical equipment, for assessing the current state of the electromechanical equipment used, timely diagnostics of emergency and pre-emergency modes of its operation, estimating the residual resource, as well as prediction the technical state on the basis of machine learning are very important.
This article is dedicated to developing the special method of data storing, collection and aggregation for definition of life-cycle resources of electromechanical equipment. This method can be used in working with big data and can allow extracting the knowledge from different data types: the plants' historical data and the factory historical data. The data of the plants contains the information about electromechanical equipment operation and the data of the factory contains the information about a production of electromechanical equipment.

Development of an Algorithm for Control Metallurgical Processes of Fluidized Roasting Using an Adaptive Controller
Дата публикации: 2018-07-19
Журнал: Journal of Physics: Conference Series
Авторы: Beloglazov, I.I, Petrov, P.A, Gorlenkov, D.V.
ISSN:17426596
In this article, we consider the solution of adaptive control problems by developing a unified algorithm for regulator tuning. The task of adaptive control of dynamic processes in real time, today plays a key role in the development of modern control systems. Existing adaptive management development assumes, as a rule, an unchanged structure of the technological process, where only the parameters of the process under investigation are subject to change. To create such systems, detailed modeling of the technological process is necessary, while the typical management model after identification becomes unique for a particular enterprise due to the parameters found. This system is designed for operating objects that allows you to take into account the dynamic change of parameters in the process of object identification while the stage of mathematical modeling is replaced by a standard approximating model.

Evaluation of bulk material behavior control method in technological units using dem. Part 2
Ключевые слова:Bulk materials | Classification of motion modes | DEM-modeling | LSTM | Neural networks | Pelletizing drums | RNN
Дата публикации: 2020-01-01
Журнал: CIS Iron and Steel Review
Авторы: Boikov, A.V, Savelev, R.V, Payor, V.A, Potapov, A.V.
ISSN:24141089
Q1
(Scimago)
The research is dedicated to the development of special devices (capsules) that can be used to control the mining ore behavior in the technological unit in order to increase processes efficiency. In the first part of the article, the choice of the discrete element method for gen-erating various particle trajectories in the unit (drum pelletizer) was substantiated. This part describes the specific technologies that were used to recognize the pelletizing mode. In par-ticular, conversation of paths to sensor readings is implemented using the Matlab Sensor Fusion and Tracking Toolbox. The obtained readings were processed using two neural network classifiers (DNN and LSTM). As a result, stable models for recognizing the pelletizing modes of the unit were obtained. LSTM recognition accuracy is greater than DNN. The developed approach can be used to recognize the operating modes of other technological units. In addition, data on particles trajectories can be used to improve DEM models of technological processes. Future work consists of the capsule physical implementation and testing the recognition algorithm on a real unit.
Отзывы партнёров
"Together with the Educational Center of Digital Technologies at St. Petersburg Mining University, we have been collaborating for several years to shape fundamental and applied challenges and ideas for the digitalisation of the mining industry."
"We are very glad to be part of the process that the Educational Center of Digital Technologies at St. Petersburg Mining University is engaged in. We are confident that this centre can become an assembly point for all those new solutions that will bring the mining industry to a new level."
The Committee for the Fuel and Energy Complex of the Leningrad Region expresses its gratitude to you for your support in holding the Festival and organising an informative exposition of the enterprise aimed at attracting the young generation to the fuel and energy complex profession.
Thanks to your efforts, we will be able to further educate young people full of strength and aspirations for knowledge and creativity in the field of energy saving.
We hope for further fruitful co-operation in the field of energy saving.
Thanks to your efforts, we will be able to further educate young people full of strength and aspirations for knowledge and creativity in the field of energy saving.
We hope for further fruitful co-operation in the field of energy saving.
On behalf of the Ministry of Energy of Russia, we would like to express our gratitude to the WeWatt team of young researchers for the great and necessary work for the industry, done under your leadership on a proactive and pro bono basis.
The results of this study will serve as a basis for further work in this area and will be useful to coal companies in carrying out digital transformation of production facilities, contributing to the effective and successful achievement of the goal.
The results of this study will serve as a basis for further work in this area and will be useful to coal companies in carrying out digital transformation of production facilities, contributing to the effective and successful achievement of the goal.
Institute for Problems of Integrated Subsoil Development, Dmitry Klebanov
Leonid Zhukov, Director of SITECH Division of Zeppelin Rusland Ltd.
Committee for Fuel and Energy Complex, Chairman of the Committee Y.V. Andreev
Ministry of Energy of the Russian Federation
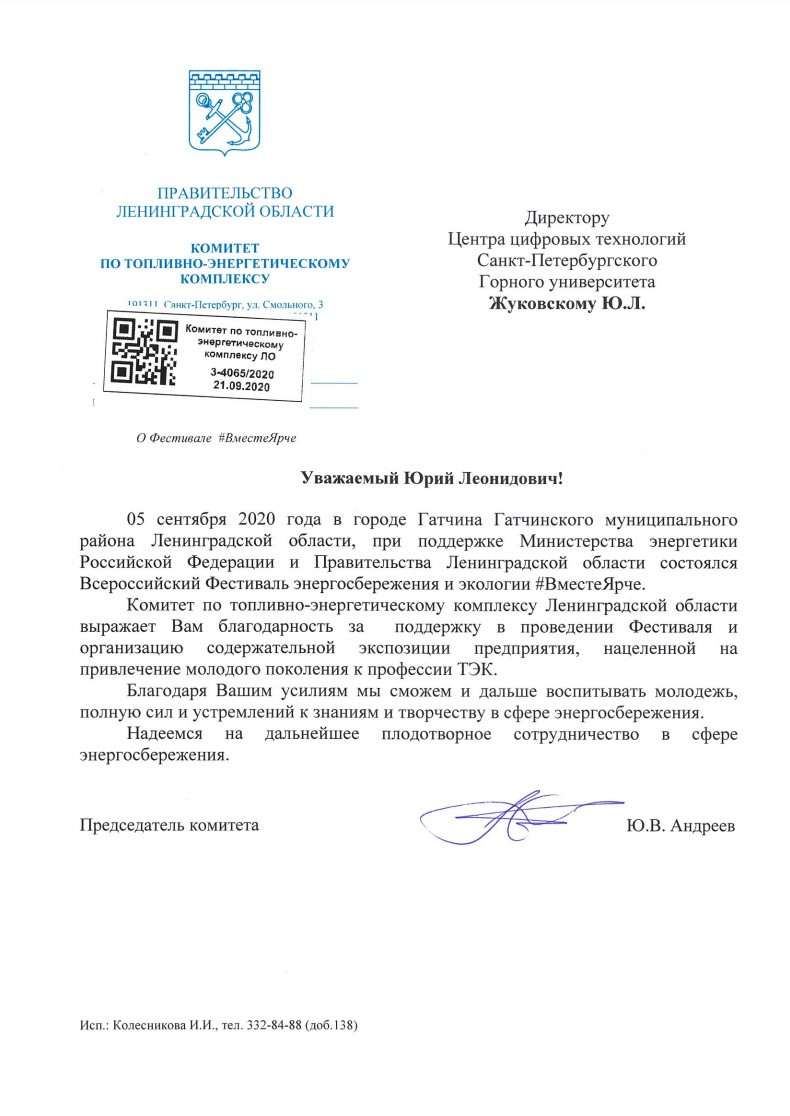
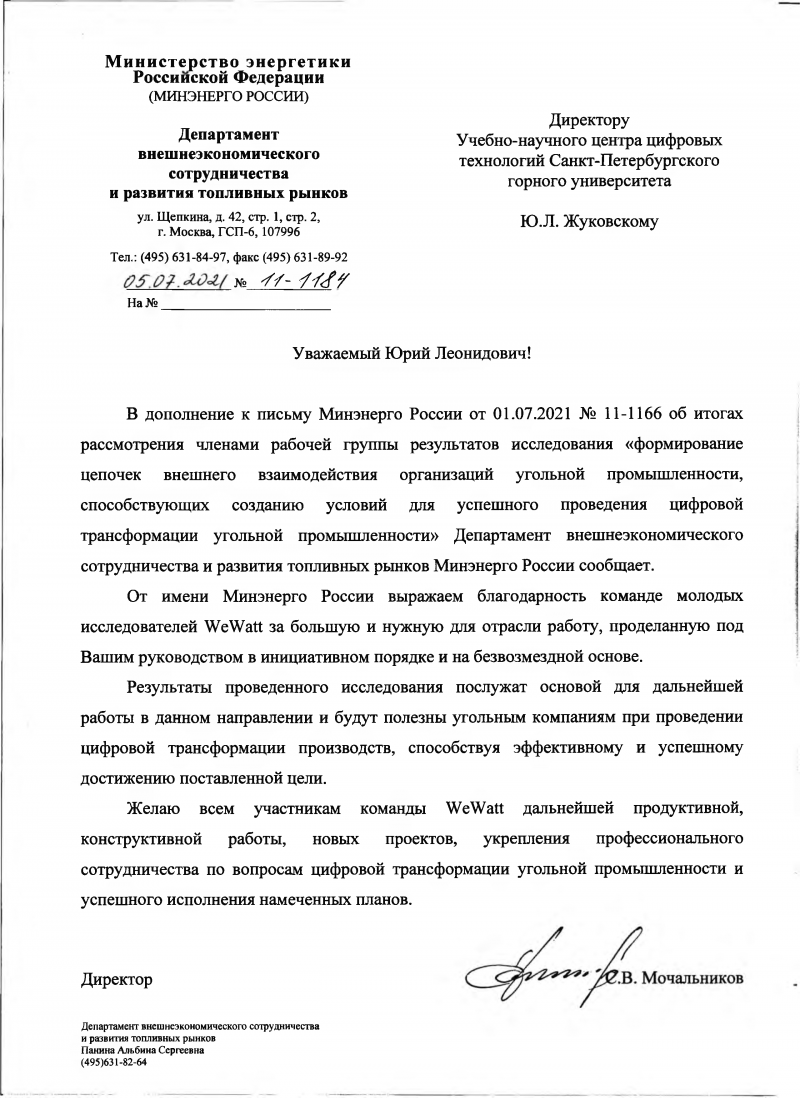
Научный центр в лицах
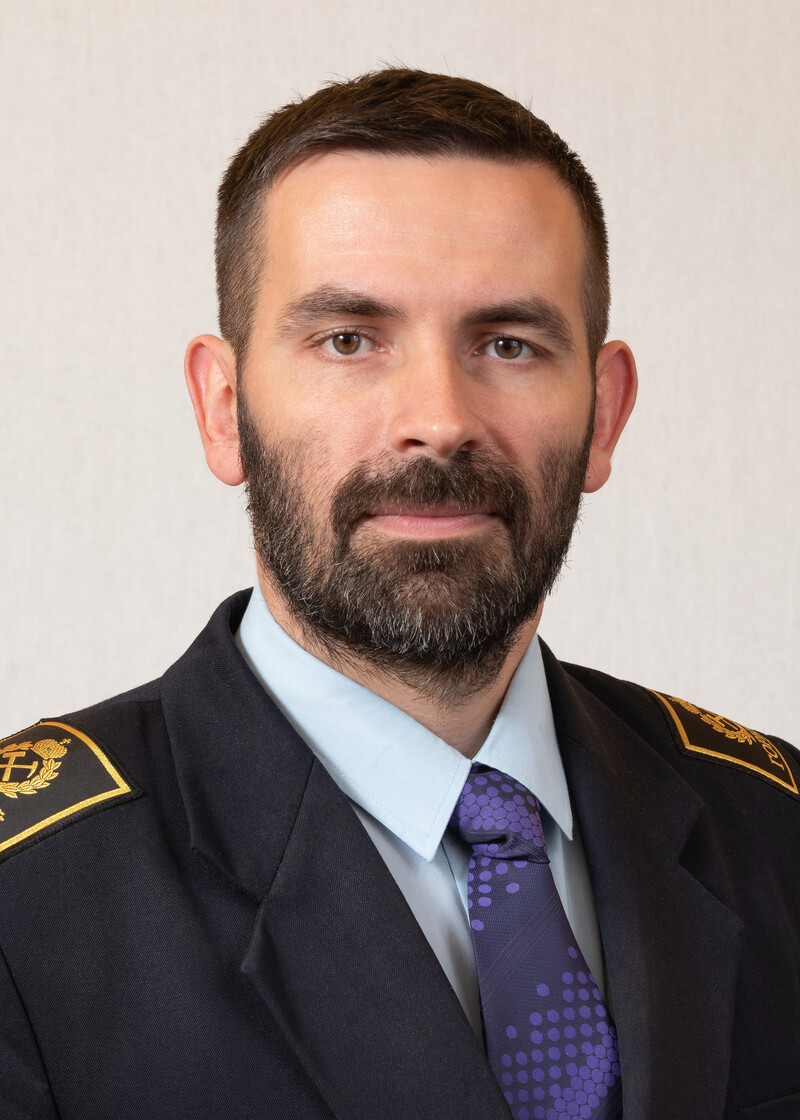
Смирнов Андрей Геннадьевич
главный специалист
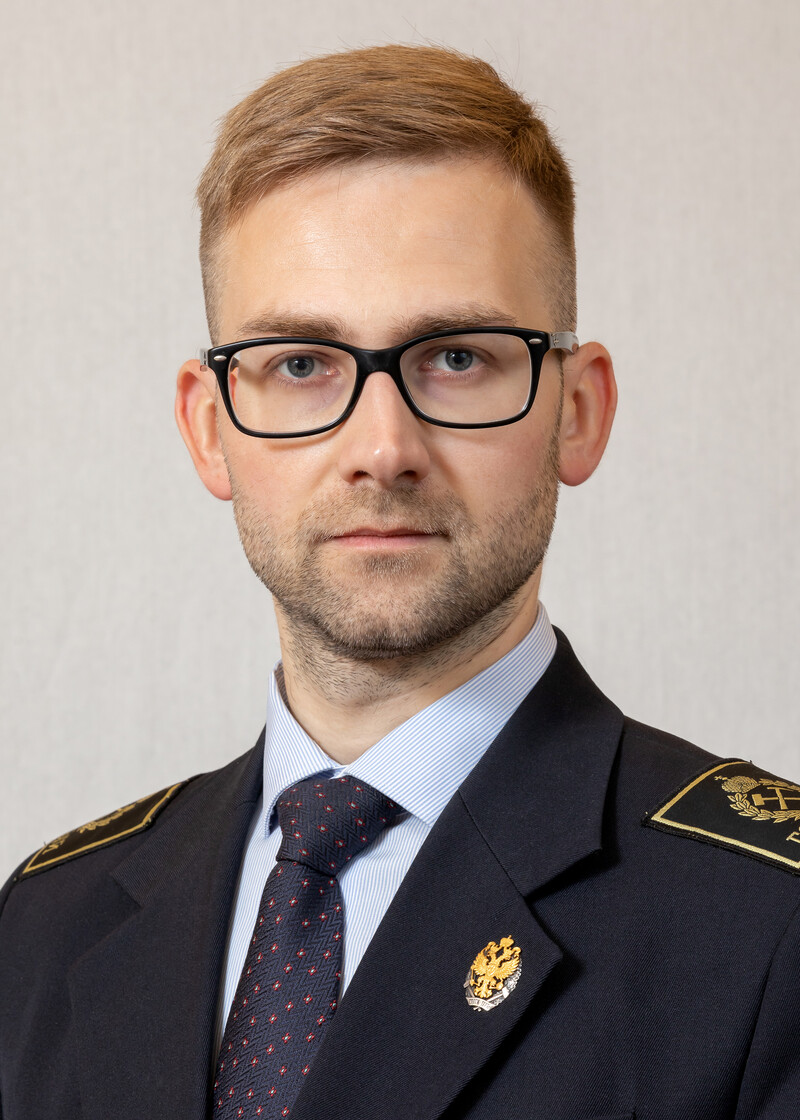
Сержан Сергей Леонидович
Заместитель директора по научно-технологической деятельности
к.т.н. / доцент
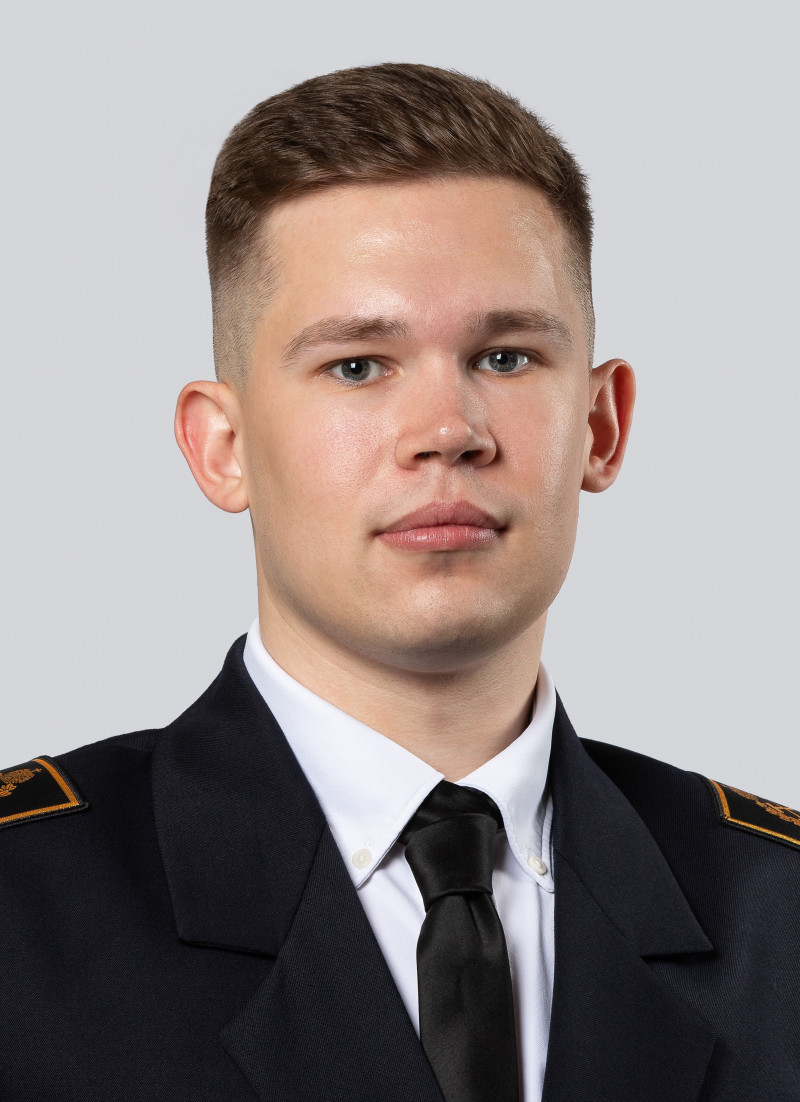
Замараев Степан Сергеевич
инженер
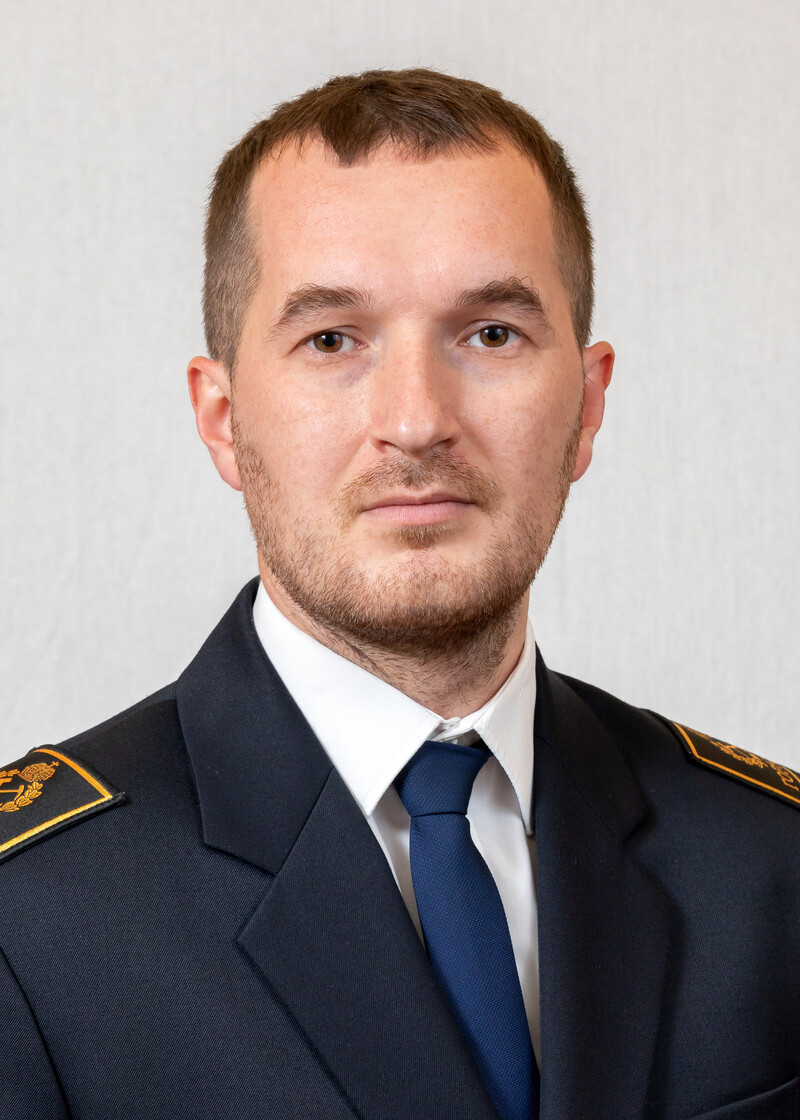
Чупин Станислав Александрович
доцент
к.т.н.
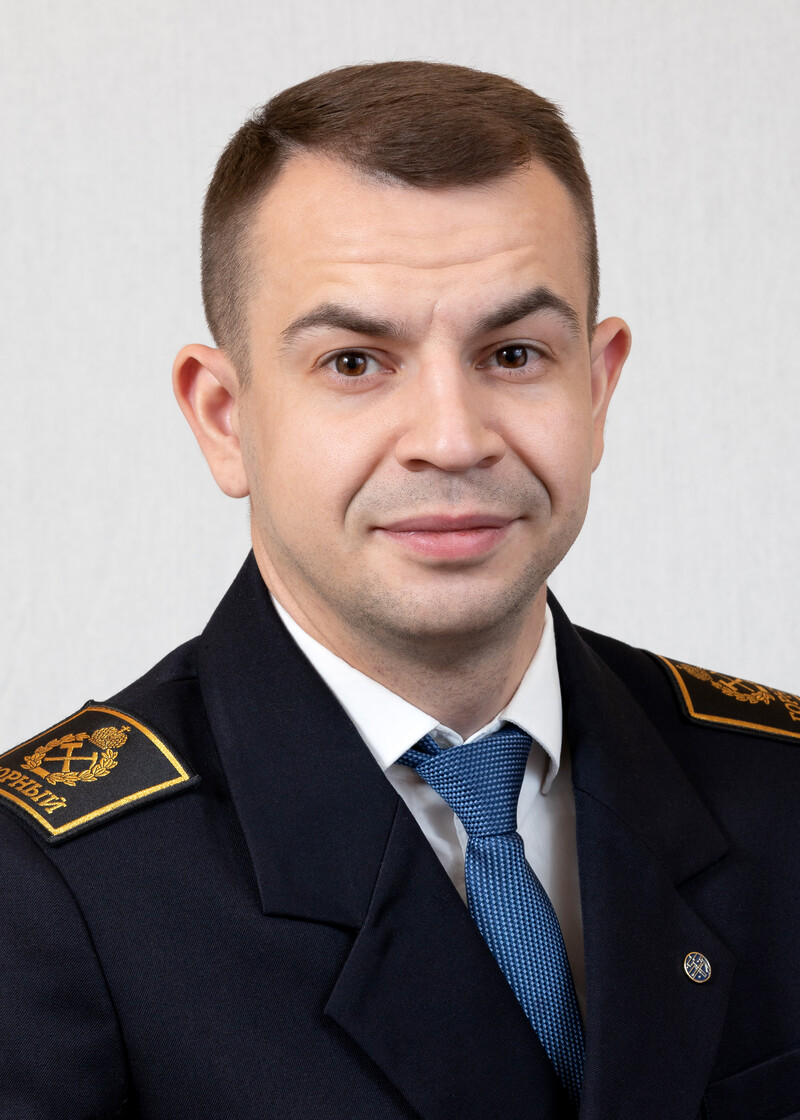
Королёв Николай Александрович
доцент
к.т.н.
Обратная связь